Project Summary
Energy is a highly required commodity of modern times. The building sector consumes nearly 75% of electricity sales. The rapid population and economic expansion, electricity consumption in commercial buildings are projected to be doubled by 2050. The building sector generates one-third of greenhouse gas, two-thirds of halo-carbon, and about one-third of black-carbon emissions. These growing energy demands have raised significant concerns around the globe regarding their adverse effect on the environment. The energy savings potential could reach as high as 30-80% with the development of smart energy management and control systems for commercial buildings. It’s extension towards traditional grids as smart grids which in turn reduces theenergy consumption and carbon emissions. Energy management is the process of tracking and optimizing energy consumption to conserve highly required IoT-based energy management and control systems that aim to provide high-quality energy by proper management of energy and maximizing energy efficiency. This system aims to be implemented for an online monitoring system and control mechanism for critical electrical equipment of the buildings such as hospitals, laboratories, and any commercial buildings.
Different sensors such as smart meters, and temperature sensors will be installed at critical electrical equipment such as transformers, photovoltaic systems (PV), overhead lines (OHL), and buildings. The sensors will then send the real-time measured data via a wireless system to the central control center (proposed at Kathmandu University Laboratory) for monitoring, controlling, and data logging. The data will be then used to display real-time critical variables such as energy loss, real-time energy production of PV systems, carbon emission reduction etc.
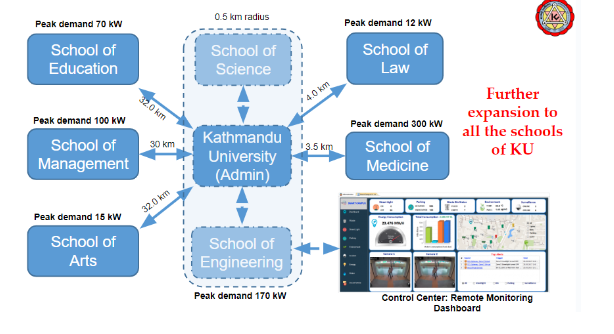
The analysis of stored data is going to be implemented as an accurate electrical forecasting tool to plan for different activities such as hour-ahead, day-ahead, yearly planning, impact and value analysis etc. By considering this necessity, a new tool for electrical forecasting of critical electrical quantities such aselectrical load demand and photovoltaic generation will be developed using advanced machine learning and deep learning algorithms (e.g. Seasonal Autoregressive Integrated Moving Average with eXogenous variables, Extreme Gradient Boosting, Prophet, Long Short Term Memory, Temporal Fusion Transformer etc.) to deal with non-linearity, uncertainties, and multiple types of inputs. The econometrics model will also be implemented to analyze the impact of different variables in the forecasting. The developed tool will be given to the electrical utilities of Nepal which will help them for better planning and decision making.
Consortium Members
Coming Soon!
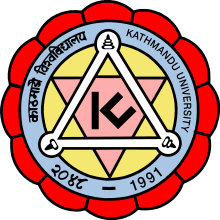
School of Engineering
Kathmandu University
Dhulikhel, Kavre